Gene Analysis Platform (GAP)
Gene Analysis Platform (GAP) for ELN
Authors: S. Merk 1, H.-U. Klein 2, M. Dugas 3, T. Haferlach 4;
1,2,3 Universitätsklinikum Münster, Germany; 4 MLL Münchner Leukämielabor GmbH, Germany Information Letter No.5, August 2008
Introduction
Gene Analysis Platform (GAP)1 was developed to provide a system for convenient data exchange between various leukemia research groups, to process large-scale microarray datasets and to assure a standardized data analysis workflow for gene profiling. At present, GAP is used by twelve EL N-workgroups covering 65 analysis sets and about 1800 microarrays.
The technical core of GAP is a SQL database running on a multi-processor linux system. This database stores all occurring data including raw and normalized data as well as analysis results. A command line interface serves as backend for data management and analysis. This back-end is implemented in R (a statistical programming language) and applies various packages of the Bioconductor project 2. Exploration of data and results is provided by a web-based front-end which is implemented in PHP (a scripting language) and can be accessed via a standard web browser.
Data exchange platform
Cooperating EL N-laboratories are spread over Europe, therefore a platform to exchange high-volume microarray data was implemented in GAP. The EL N file manager enables transfer of raw data, for instance Affymetrix cel-files, as well as files containing clinical/phenotype information.
Data preprocessing and analysis
Microarray data preprocessing consists of several steps: parsing of raw data, normalization, quality assessment and filtering. Raw data can be imported by GAP in various formats, for example as Affymetrix cel-files or in tabular format. RMA, VSN and MAS5 2 are implemented for normalization of raw data. A comprehensive quality check includes assessing raw data chip images, differential chip images, 3'/5'-ratios of housekeeping genes like ß-Actin or GAPDH, boxplots or density plots as well as Affymetrix specific quality parameters to identify chips with poor quality. Unspecific filtering helps to exclude genes that are likely to be non-expressed in the respective cells or tissue. It is also possible to filter out genes with low variance across all samples.
Several methods are implemented to reveal structure in the data without including class label information. Principal component analysis PCA) is a standard technique to analyze multidimensional datasets. PCA can be visualized as 2Dor 3D-plots. Hierarchical clustering provides
a partitioning of data into subsets according to their similarity. Clusters can be visualized with trees (dendrograms). Heatmaps with color-coded expression values can be generated to visualize microarray data.
A very common analysis method is identification of differentially expressed genes between different disease subgroups. The goal is to identify specifically up- or down-regulated genes. GAP provides methods like t-test for twogroup comparisons as well as analysis of variance (ANOVA) for comparisons of more than two groups. Various statistical parameters can be calculated, such as fold change, false discovery rate according to Benjamini & Hochberg or Storey & Tibshirani) and family-wise error rate according to Bonferroni (Fig. 1).
Fig. 1: A screenshot of GAP, showing results of differentially expressed genes.
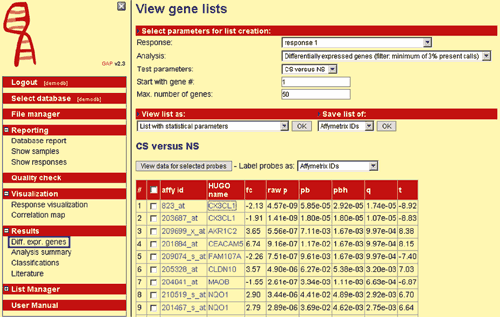
GAP also provides various methods for class prediction. Support vector machine algorithms can be applied to a training set to establish a prediction rule for an independent test set. These methods were applied to large leukemia microarray datasets 3. A new feature of GAP are transcriptome correlation maps 4 which can be used to identify regions of adjacent genes showing correlated gene expression profiles. From our experience, consistent biomedical interpretation of gene lists can be a challenging task, therefore we are developing the leukemia gene list web service (LGWS). It enables a systematic comparison of gene expression data with published leukemia gene lists. After input of gene expression data and a clinical variable of interest, these data are compared with published gene lists. Feedback from EL N members about relevant publications regarding leukemia gene lists to be included in LGWS is highly appreciated.
Outlook
Currently we are working to provide methods for integrated analysis and visualization of gene profiling data with other chip platforms, in particular ChIPchips, SNP chips and microRNA chips 5. All EL N members are welcome to use GAP, which is available via workpackage 13. For more information or a demo account please contact: dugas@uni-muenster.de.
References
- Dugas M, Weninger F, Merk S, Kohlmann A, Haferlach T. A Generic Concept for Large-scale Microarray Analysis Dedicated to Medical Diagnostics. Methods Inf Med. 2006;45:146-152
- Bioconductor Project. [http://www.bioconductor.org] 3. Haferlach T, Kohlmann A, Schnittger S, Dugas M, Hiddemann W, Kern W, Schoch C. Global approach to the diagnosis of leukemia using gene expression profiling. Blood 2005;106:1189-98
- Reyal F, Stransky N, Bernard-Pierrot I, et al. Visualizing Chromosomes as Transcriptome Correlation Maps: Evidence of Chromosomal
- Domains Containing Co-expressed Genes—A Study of 130 Invasive Ductal Breast Carcinomas. Cancer Research 2005; 65: 1376-1383
- Isken F, Steffen B, Merk S, Dugas M, Markus B, Tidow N, Zühlsdorf M, Illmer T, Thiede C, Berdel WE, Serve H, Müller-Tidow C. Identification of acute myeloid leukaemia associated microRNA expression patterns. Br J Haematol. 2008;140:153-61 [Supported by: European LeukemiaNet, WP 11 and WP13, and Rolf Dierichs-Stiftung]